The Grand Challenge ahead is to shift fossil-dominated centralized energy systems towards regenerative integrated multi-vector grids. This requires also sustainable electrical energy storage, including the related raw material supply, processes and systems. A real impact on economy, society and ecology is only created if materials, processes and products can be potentially transferred to large scale. This represents a particular challenge for mid to long term, systems-integrated energy storage, also because the EU strongly depends on critical raw materials from politically instable regions. In VanillaFlow, we develop radically new approaches for integrated energy storage which combine artificial intelligence (AI) and machine learning (ML) with flow battery technology to replace currently employed, non-sustainable, and critical raw materials (i.e. redox-active molecules, membranes) in flow batteries by readily-available renewable materials based on starch and lignocellulosics. VanillaFlow will use AI and ML techniques such as physics-informed modeling, causal discovery, and representation learning, and makes use of deep learning and symbolic regression. These approaches are used in designing redox active quinones, and to optimize their interplay with the other components of a battery on single and multi-cell level. The whole research will be guided by toxicology investigations to ensure that sustainable and inherently safe materials will be obtained in the project. Today, the innovation capacity of European scientists and industry in the area of renewable materials makes them already the leading global players in the field. VanillaFlow will further support the European technological leadership in the area by cross-fertilization of different fields (artificial intelligence, battery technology, pulp and paper, biotechnology, polymer technology, toxicity) while addressing needs of sustainable materials in mid to long term energy storage.
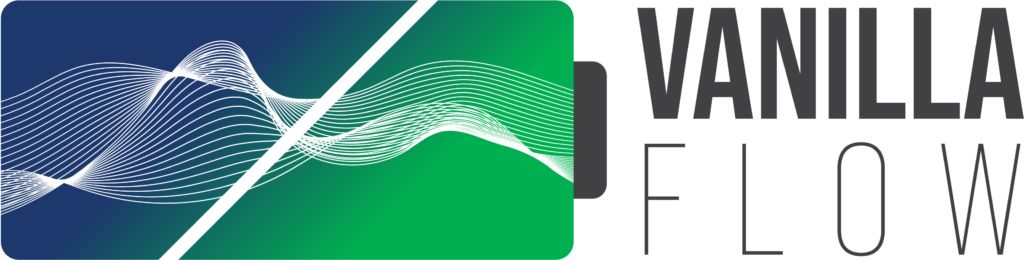
Artificial Intelligence Guided Development of Vanillin-based Flow Batteries
This Project has received funding from the European Union’s Horizon Europe Research and Innovation programme under the grant agreement No. 101115293